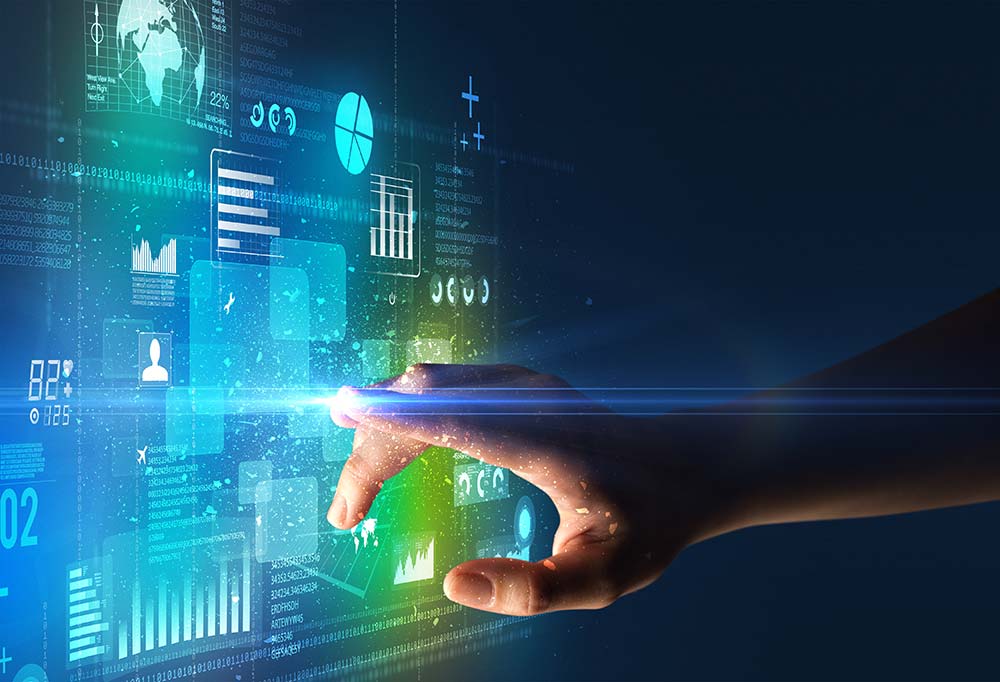
This article first appeared in National Defense Magazine on 5/20/2019.
As the United States faces threats that are more complex and rapidly evolving than ever, the Defense Department’s technological arsenal must be ready to face any challenge.
Just as the dramatic innovation of info-tech systems and software capabilities have revolutionized the military’s ability to execute its mission, successful formulation and implementation of an artificial intelligence strategy is critical to the success of the next-generation military.
The AI arms race is already underway amongst the United States, Russia, China and other more technologically advanced nations. In order to succeed in the global arena, the Pentagon must continue to make strategic investments to deploy AI systems that will strengthen the military and support the warfighter.
The Defense Department must work with industry partners, including large contractors and startups who have the right capabilities and domain expertise, to harness the power of modern AI and further develop and tailor this technology to meet the unique and constantly evolving challenges faced by today’s modern military. The AI systems must be robust, secure and explainable so they can perform as intended in a way that is consistent with the values of the nation.
Artificial intelligence has delivered on a number of its promises through advancements made in the past few years. Driverless cars, natural language translation, virtual assistants, and robots in homes, stores, factories and on the battlefield are just a few examples of this trend. The “AI winter” has ended — the success of AI methods has restored confidence in the approach, and funding for the field has increased in recent years in both the private and public sectors.
Several applications of the technology the Defense Department is working on are either already being explored or are within reach. These include automatic target recognition in intelligence, surveillance and reconnaissance, optimal resource allocation, cost reduction, improved maintenance scheduling for aircraft, vehicles, etc., physical and cyber threat detection, logistics and transportation, health care, training, combat simulations, human capital, energy consumption and data compression.
Applying AI methods to these problems requires leveraging a combination of structured and unstructured data. As an example, logistics data from structured databases as well as onboard vehicle sensors — likely unstructured — can be mined to learn patterns, optimize routes and predict when vehicle maintenance will be required. Machine learning methods, such as neural networks, can be applied to unstructured aerial videos and photographs to detect threats or targets of interest. Many videos and photographs with features representative of real-world scenarios would need to be collected to train algorithms so they can make reliable predictions.
Other federal agencies have embraced AI solutions as well. The CIA is conducting pilot projects and highly values the ability to tie correlations from data to cause and effect.
The Department of Homeland Security, IRS, General Services Administration and NASA are just a few of the many other organizations that have begun to reap the benefits of implementing AI solutions. The Defense Department can learn from these efforts, apply and tailor best practices to its use cases and mission.
Artificial intelligence is critical for the Defense Department’s success because of the unique challenges it faces. Missions and operations are extremely complex and often require real-time, high quality information and insights in order to succeed. The department relies on a complex, massive, decentralized network of both internal and external data sources with a wide variety of data types and formats. Adversaries continuously pose new and more evolving threats, both physical and cyber.
For the military, “big data” is not just a buzzword. Being able to effectively and rapidly collect, cleanse, organize, sift through, analyze, safeguard and utilize enormous volumes of diverse data is critical to operational and mission success. These data sources include not only data owned by the Defense Department, but also public data, information provided by other federal agencies, contractors and partners. Humans alone are not able to utilize these data sources and streams to their full potential, especially in real-time situations where every second counts.
By using AI, warfighters and combatant commanders can have maximum situational awareness, allowing them to better protect themselves and civilians, intercept threats, and improve their ability to deliver results, enhance readiness, eliminate threats and execute on the mission.
The technology is not yet at the point where it can simply be plugged in to solve any problem. Customization is required and depends heavily on the particular data being used to train models. Experience on both the technical and domain-specific fronts is required for success. Large contractors and startups who have solved similar challenges for commercial clients and have experience working for the Defense Department and other federal agencies are ideally suited to tackle these large-scale enterprise challenges. They are able to translate the department’s strategic questions into mathematical models that can be solved by carefully analyzing data using the best tools available and evaluating results in the unique context of the military’s missions.
Although the time is ripe for the Defense Department to use AI, such efforts will fail without a strong strategy and implementation plan. Domain expertise should be used at every stage of the process, rather than only relying on data. The goals and performance metrics of an AI system must be clearly defined at the outset. Then, the appropriate data must be collected and organized. Data must be processed appropriately so that AI algorithms can be used to extract insights.
Algorithm selection, tuning and customization are critical for building robust models. Results should be validated and compared to key performance indicators. Multiple rounds of iteration of data processing, model development and validation are usually required. Once data scientists are confident in the model they have developed, data can be used for analysis, business intelligence, visualization, or tool development, depending upon the use case.
AI system construction and deployment does not exist in a vacuum, and software development and database administration best practices should be followed. Networks, databases and data streams must be secured and rated at the appropriate classification level. Data governance policies and tools should be used to maintain data integrity across and among organizations.
Other considerations include storage capacity, processing power and cloud capabilities. Supporting software to ingest data, run models and handle outputs must be developed and integrated with AI software. All software should be developed using modern proven approaches, such as Agile and DevOps, to ensure high quality and scalability. Training data to be used in machine learning or other AI algorithms may need to be manually labeled or processed. Databases must be designed in a flexible, extensible manner to enable data to be sliced as needed for analysis and utilization.
Pilot projects should be executed to rapidly prototype solutions for simpler versions of the problem at hand to assess feasibility of the approach relative to the key performance indicators. If the results of the pilots are trending in the direction of meeting target metrics, then progressively larger pilots and rollouts should be deployed. Hackathons, or innovation challenge events, are a good example of the rapid prototyping process. These types of events have become widely used in the private sector to develop and apply AI algorithms to solve problems. The Defense Department and many other federal agencies have hosted numerous hackathons and similar events — including non-sensitive events open to the public — to encourage experimentation with new applications of data science and AI to their organizations’ challenges.
By breaking down silos within and across the Pentagon and partner organizations, data can be shared and utilized more effectively and provide mutual benefits. Contractors who have run hackathons for private sector firms can help the Defense Department expand the use of such events to benefit the military.
Many of the same challenges that the private sector faces in applying AI are also relevant for the Defense Department. One drawback of many machine learning techniques is the so-called “black box problem.” Although these algorithms can detect patterns effectively and with high accuracy, it can be difficult to explain their internal logic. This poses a challenge for the Defense Department, which requires insight into how algorithms are operating for performance and ethical reasons.
Machine learning algorithms also require large, granular, high-quality data sets in order to learn patterns. They must be retrained as the situation to which they are being applied evolves. While machine learning has proved useful for solving many complex big data problems, other AI approaches may be required in addition to, or instead of, machine learning.
The Defense Advanced Research Projects Agency refers to “contextual adaptation” as the “Third Wave” of AI. This is also known as causal inference or system dynamics.
While early AI approaches were based on listing out rules, the First Wave, and machine learning uses statistics to find patterns without any knowledge of a system, the Second Wave, system dynamics involves constructing a model based on domain knowledge, available data, and human intuition to produce detailed, easily explainable models that can be used to make decisions.
Models developed using this approach reveal the causal relationships among relevant factors, while most machine learning methods can only calculate correlations between inputs and outputs. System dynamics can directly answer both strategic and more granular queries, while the results of a machine learning analysis tend to answer more targeted tactical questions. Even when available data is limited or unstructured, system dynamics can produce useful results.
Comprehensive, adaptable models of systems are key for improving the Defense Department’s ability to make effective decisions in a timely manner. AI solutions are needed to supplement human intuition and expertise in order to quickly process massive amounts of varied data to gain useful insights.
As threats become more sophisticated, mission success will depend on executing a flexible, evolving AI strategy with help from industry partners whose tools and techniques can be tailored to the complex environments in which the military must operate.
James B. Marceau and Barry E. Scharfman, Ph.D. are aerospace and defense experts at PA Aerospace and Defense, a subsidiary of PA Consulting Group.